Continuous Behavior Acquisition in Clinical Environments
K.J. Chen, “Continuous Behavior Acquisition in Clinical Environments,” University of California San Diego, 2018.
Abstract
Continuous behavioral labels of hospital patients provide quantitative data that can be informative for both research studies and clinical applications. An analysis of neural correlates to natural behavioral labels extracted from pose estimates, for example, could enable more robust brain-machine prostheses for those with limb loss or motor impairment. Automated patient motion analysis could also provide additional insight to clinicians during motor scoring assessments and seizure classification for a better-informed diagnosis. Likewise, continuous patient safety monitoring enabled by posture annotations could detect potential bed falls or other injury risks and quickly alert nurses to administer preventive measures. Such scenarios rely on consistent and accurate patient posture tracking in clinical environments.
While many existing pose estimation frameworks are effective when subjects are located in uncluttered settings, clinical environments can provide several visual challenges that these general frameworks are not calibrated for. In this thesis, we propose a semi-automated approach for improving upper-body pose estimation in noisy clinical environments, whereby we adapt and build around an existing joint tracking framework to improve its robustness to environmental uncertainties. The proposed framework uses subject-specific convolutional neural network (CNN) models trained on a subset of a patient’s RGB video recording chosen to maximize the feature variance of each joint. Furthermore, by compensating for scene lighting changes and by refining the predicted joint trajectories through a Kalman filter with fitted noise parameters, the expanded framework can yield more consistent posture annotations in these settings when compared to general methods. The perspectives gained from this work provide better insight in developing a practical pose estimation framework for researchers and clinicians in these environments.
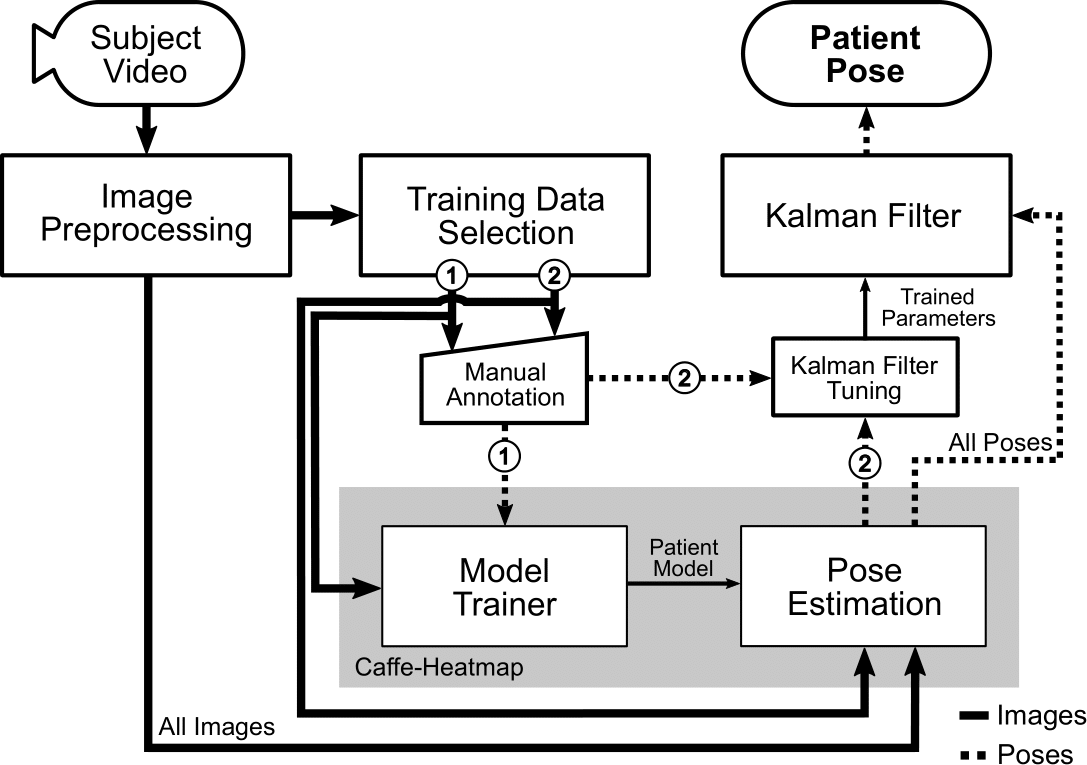
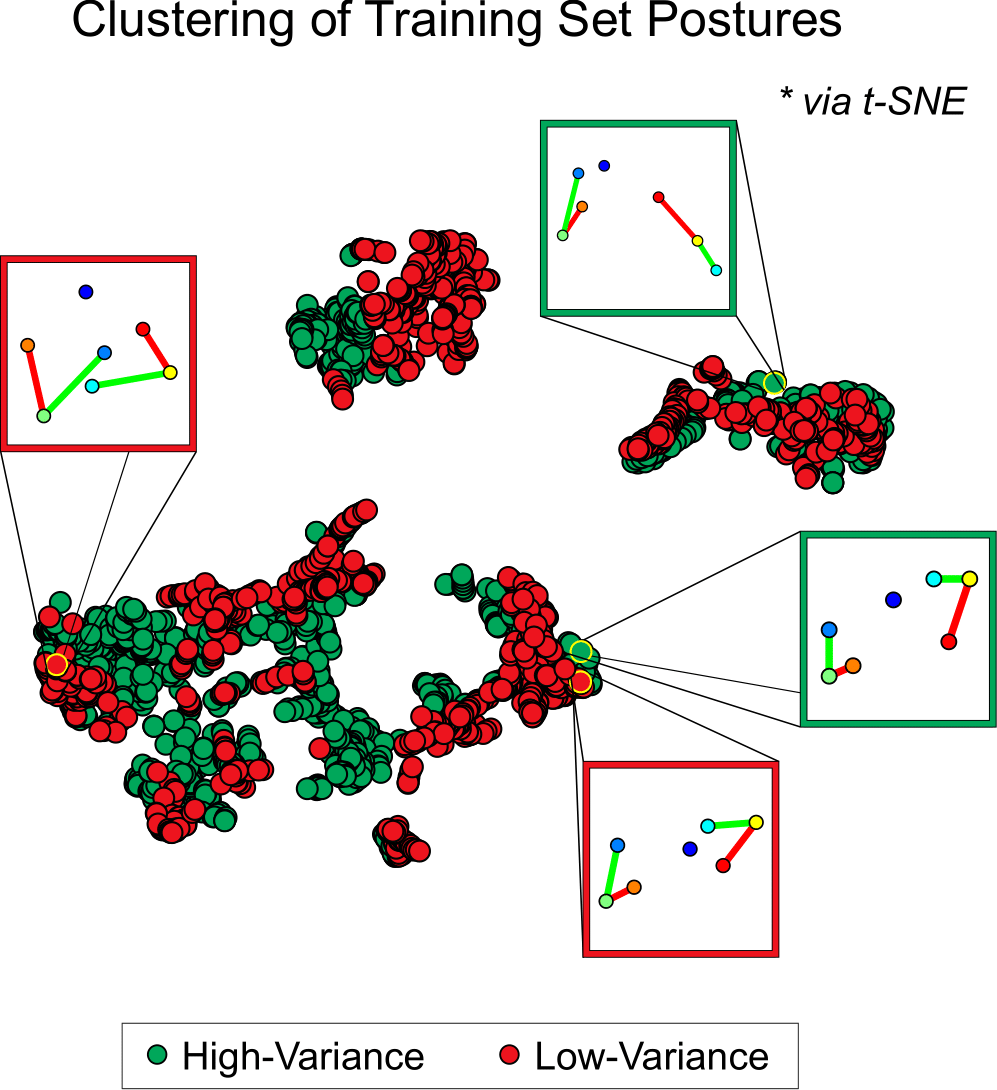